Hi! PARIS Fellowship 2023 – David Restrepo-Amariles
Hi! PARIS is pleased to announce its new Hi! PARIS Fellow in Law, David Restrepo Amariles.
We are glad to announce that David Restrepo Amariles, associate professor of Law at HEC Paris, has been awarded a Hi! PARIS Fellowship for his project on cross-Jurisdictional AI methods for Civil Court Law decisions.
David’s proposal builds from the fact that existing AI models in civil law jurisdictions were actually developed by adapting methods originally used for common law jurisdictions.
The chief goal of the project is to fill this gap in current AI, and to develop multi-lingual AI methods that can be transferred across civil law jurisdictions. To that objective, the project will be conducted in collaboration with the European partners of the Smart Law Hub.
Cross-jurisdictional AI Methods for Civil Law Court Decisions
This project seeks to fill the gap in the legal domain is a key modern challenge, as evidenced by the fact that the European Union’s mooted AI Act explicitly considers such use as “high risk”. Yet, the development of AI for adjudication remains, in fact, under-developed, partly because research on and development of AI methods and tools in this domain remains expensive, data-intensive, and politically sensitive. In addition, language barriers decrease the potential benefits of using AI models trained on a single jurisdiction to other legal contexts.
This project seeks to fill the gap in current AI and legal research by focusing on the development of models fit for AI implementation in civil law jurisdictions. While civil law jurisdictions represent a majority of the world’s legal systems (Graph 1), and share significant characteristics, the majority of AI tools available in civil law jurisdictions are based on methods and models development in common law jurisdictions (including common law datasets) that are retrained and transposed to civil law jurisdictions.
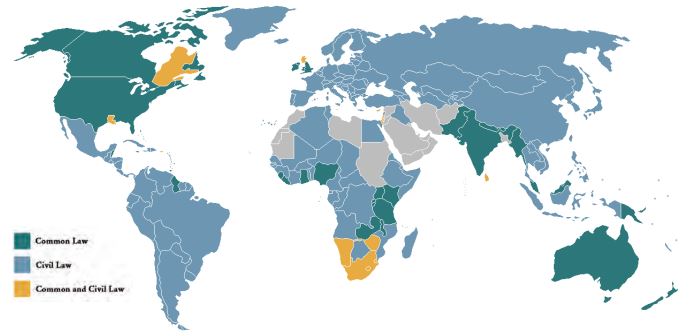
Civil law jurisdictions share historical and doctrinal and philosophical roots, and are characterized by broadly similar features that have not been capitalized to date in research. These include an emphasis on legislative norms (as opposed to customary law and precedent); a mix of inquisitive and adversarial proceedings; a strong role reserved for the judge (as opposed, e.g., to jury-based fact determination); a highly-formalized style of judgment centred around syllogisms (as opposed to common law narratives); and a language and formulae specific to legal pleadings and concepts. As a result, civil court judgments and approaches to the law tend to be similar, regardless of the court’s seat or the language of the proceedings.
In this context, we examine the possibility of developing multilingual AI methods that can be transferred and generalized across civil jurisdictions to leverage the advances achieved in data-rich environments to data-poor ones. Recent research has evidenced that language models, especially when fine-tuned to be domain and/or language specific, can achieve strong performances on a variety of tasks that were once believed to be the sole remit of legal professionals. Such models, however, often rely on substantial amounts of data, and are thus presumably ill-fit for jurisdictions where the data is either unavailable and/or the available dataset is too small, and not expected to grow significantly (e.g., paper-based developing countries). What these jurisdictions have in common, however, is often a language with another, data-rich jurisdiction, and roots in a legal family, such as civil law. For instance, Liechtenstein’s relatively-small jurisprudence is all in German, and of the civil-law type; while training a language model on the Principality’s legal dataset would be challenging, it should be possible to rely on the (broader) case law of its German-speaking European neighbors.
In this light, the project starts with an exploratory study on a multilingual dataset from Belgian court decisions to examine the possibility of transferring and generalizing models developed in that jurisdiction to other jurisdictions sharing the same languages and civil law tradition. The models will be tested, and if needed retrained, with datasets made up of court decisions from Germany, the Netherlands and France. Belgian courts decisions represent an optimal dataset, as the state’s jurisprudence is both broad and multi-lingual: the resulting models, based on Dutch, French, and German court judgments, will then more easily be checked and benchmarked against that of its neighbors, namely the Netherlands, France, and Germany.
To assess the promises (and limitations) of this approach, we explore four main tasks, which have been at the forefront of the use of artificial intelligence in the judicial domain: anonymization, argument mining, predictive models, and legal explainability (i.e., justification of decision by legal reasoning). These tasks are characterized by a high reliance on written, text data, in a way that is meant to associate textual symbols (e.g., the court’s reasoning) to an outcome – making them ripe for computer-assisted analysis. These tasks are also, to some extent interrelated: detecting arguments helps in understanding roles in a judgment, which in turn informs anonymization (e.g., where only claimants need to be anonymized, but not judges), predictive justice (to identify the determinants of a given case), and legal explainability.
As such, it will be essential to identify what kind of model (fine-tuned to a particular task or generalist) performs the best, and in what conditions. In this light, the research proposal will focus on testing the model developed in several contexts, and checked against several benchmarks, be they off-the-shelf or designed specifically for this research endeavor.
David Restrepo Amariles
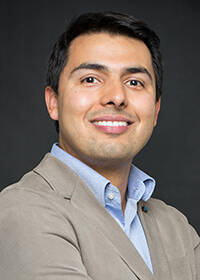
David Restrepo Amariles is Associate Professor of Data Law and AI at HEC Paris and holds the Wordline Chair on the Future of Money. He is a Member of the Royal Academy of Sciences, Letters and Fine Arts of Belgium, where he sits at the Collegium and the Technology and Society Class. He is the Director of the SMART Law Hub — Scientific Mathematical, Algorithmic, Risk and Technology driven law and Project Lead of the Smart Contracts and Regulatory Technologies project at the Cyberjustice Lab.
David teaches courses on TechLaw, Data Analytics and AI, The Future of Money and Digital Assets, and Data Governance and Compliance.