Summer School – Program & Speakers
- Home
- Summer School – Program & Speakers
Learn about this year’s Summer School program & speakers
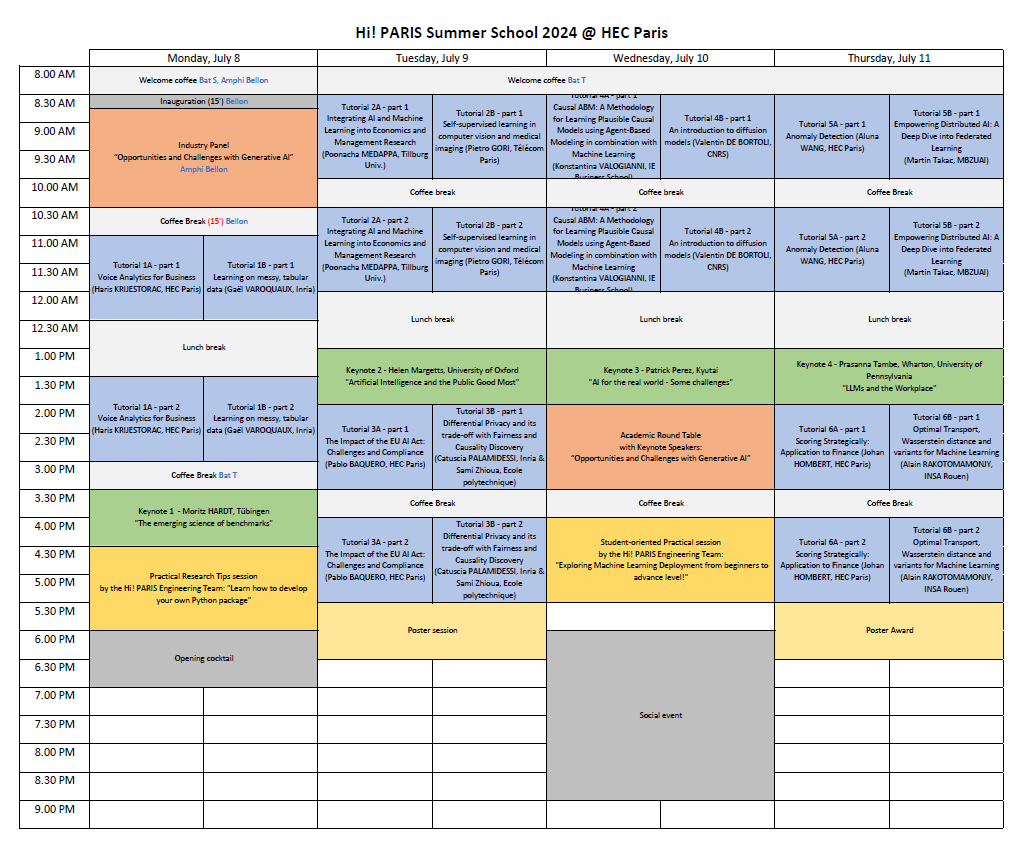
Tutorials
This year’s Hi! PARIS Summer School tutorials are meant to teach participants the best programming practices in AI and Machine Learning. Tutorials will be organized in two parallel tracks, Track A – Data Science for Business and Society and Track B – Theory and Methods of AI.
Both tracks will be held simultaneously during the week and participants will be able to choose between both tracks for each of the six tutorials.
More information on each tutorial can be found below.
Track A – Data Science for Business and Society
Tutorial 1A - "Voice Analytics for Business"
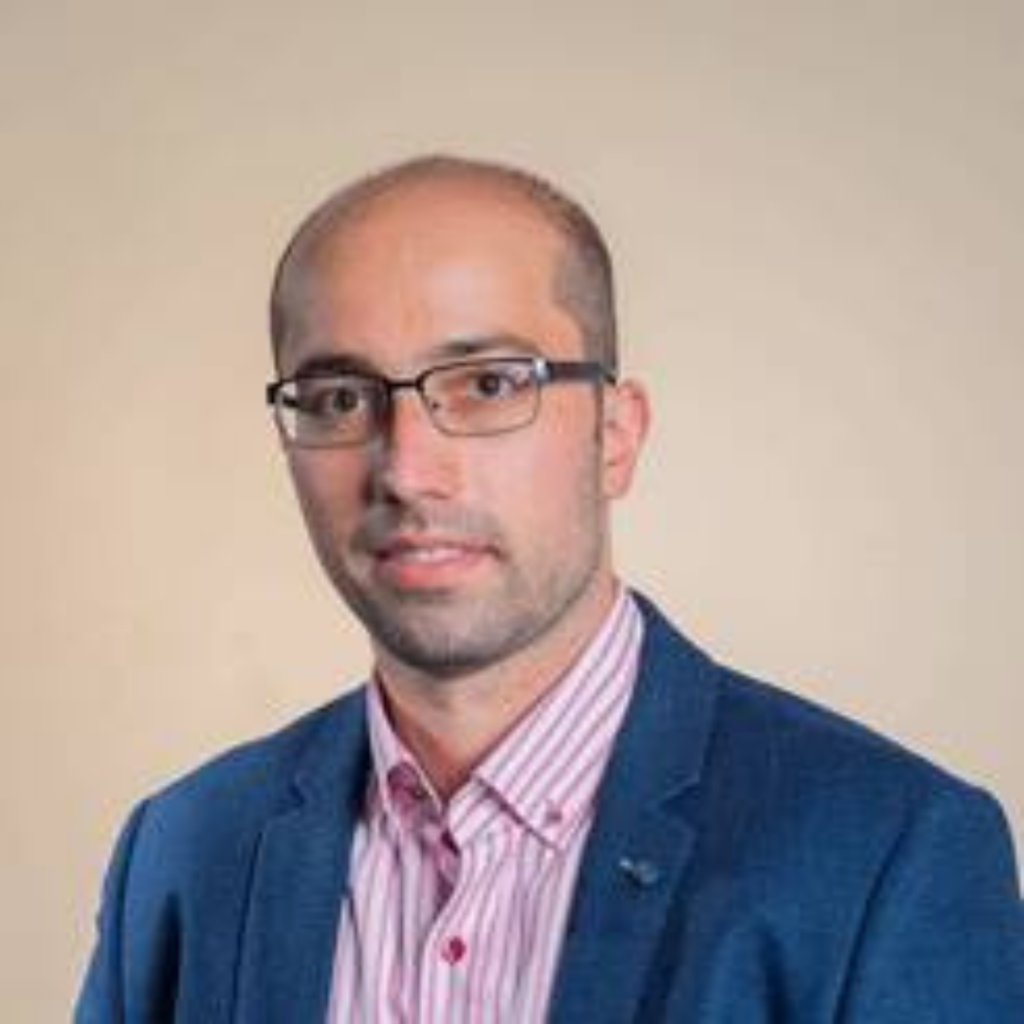
July 8, 2024 | 11:00 AM – 12:30 AM (part 1) & 1:30 PM – 3:00 PM (part 2)
Abstract: With the rise of voice-enabled digital interfaces, including Siri and Alexa, firms have growing interest in understanding how characteristics of a voice may affect business outcomes, such as engagement or sales. Drawing from the physics of sound, we will discuss the key acoustic properties of voices – including pitch, volume, harmonics, and tempo – with demonstrations on how to extract these features from voice clips. We will illustrate how to holistically analyze these acoustic features in the context of voice, through an example of research on the role of voice in consumer decision-making. Session attendees will have an opportunity to conduct similar analysis using a voice dataset, from which they will extract business insights.
Tutorial 2A - "Integrating AI and Machine Learning into Economics and Management Research"
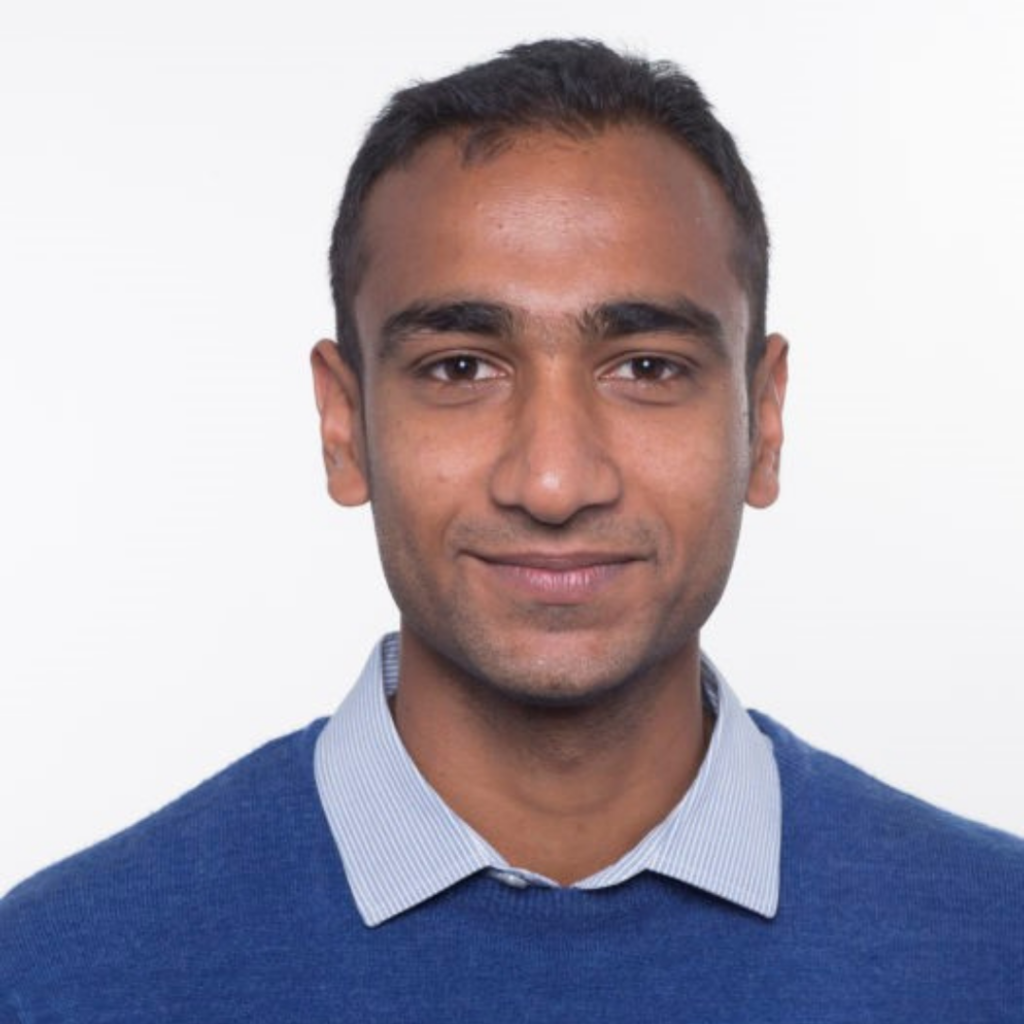
July 9, 2024 | 8:30 AM – 10:00 AM (part 1) & 10:30 AM – 12:00 AM (part 2)
Abstract: The capabilities of machines are advancing rapidly, with examples such as ChatGPT’s human-like reasoning and creativity, Copilot’s capacity to become our peer-programmers, Facebook’s facial recognition technology, and Google’s new AI and ML frameworks like Tensorflow. With these advancements, researchers now have a large toolset of approaches to perform data-driven research and provide insights that were previously infeasible. But, as researchers, how will these advancements change our research identity and the nature of our research? For instance, face recognition algorithms do not follow predetermined rules for detecting certain pixel combinations that make up a face, based on human understanding. Instead, these algorithms utilize a vast dataset of labeled photos to estimate a function f (x), which predicts the presence y of a face based on pixels x. This approach has similarities to econometrics and raises important questions, which we will address in this workshop. Specifically, we will answer three questions – (a) Are these algorithms simply utilizing conventional methods to process extensive and innovative datasets? (b) If these are new empirical tools, how do they relate to existing knowledge? and, (c) How can we as researchers incorporate these methods into our own research?
Tutorial 3A - "The Impact of the EU AI Act: Challenges and Compliance"
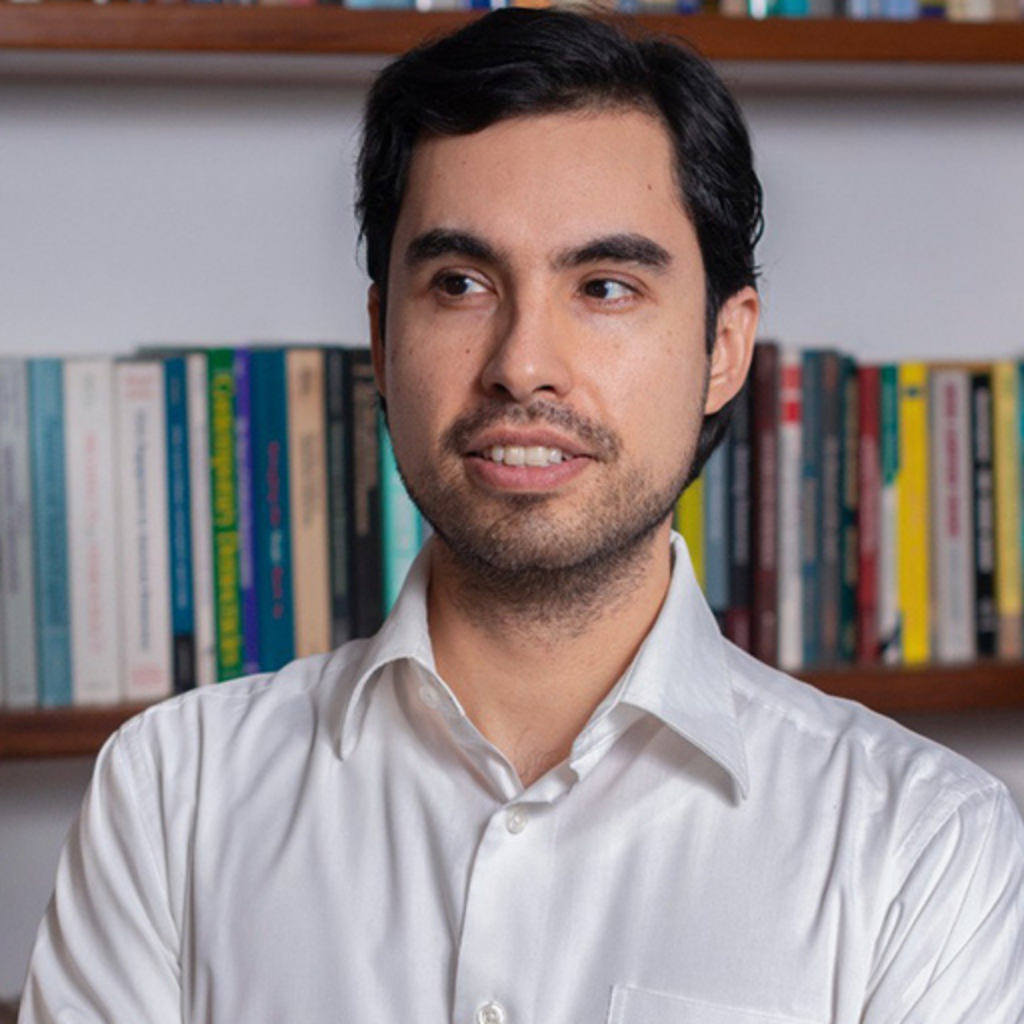
July 9, 2024 | 2:00 PM – 3:30 PM (part 1) & 4:00 PM – 5:30 PM (part 2)
Abstract: This workshop will examine how the recently adopted European AI Act will impact artificial intelligence applications to be launched in the market and the applicable requirements to comply with it. What products and services are considered as artificial intelligence under the future European AI Regulation? And how to classify AI applications under the different levels of risk established by the Regulation, which determine the rules applicable to them? Departing from these questions, this tutorial will examine in-depth two case studies involving high-risk AI applications, which must fulfil different requirements. Most of these general requirements established by the Regulation (e.g., transparency, explainability, fairness, robustness, accuracy) are further specified by technical standards stipulated by European standardization organizations for specific contexts. We will compare the risk management system created by the EU AI Act with the voluntary NIST AI Risk Management Framework prevailing in the US and discuss whether and to what extent the AI Act creates a regulatory model that will expand beyond Europe and that can effectively strike a balance between regulation and innovation.
Prerequisites:
Tutorial 4A - "Causal ABM: A Methodology for Learning Plausible Causal Models using Agent-Based Modeling in combination with Machine Learning"
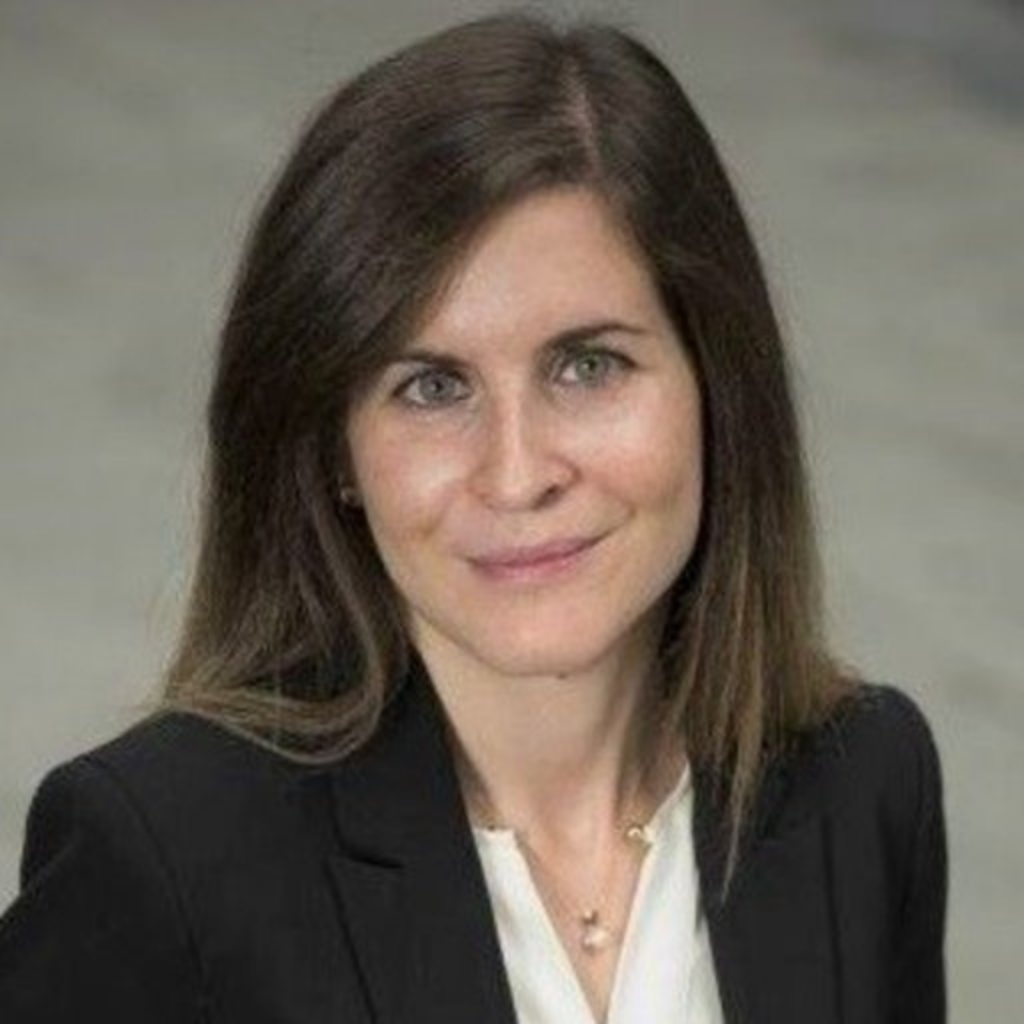
July 10, 2024 | 8:30 AM – 10:00 AM (part 1) & 10:30 AM – 12:00 AM (part 2)
Abstract: This tutorial will present Causal ABM in combination with Machine Learning (ML), a methodology to derive causal structures describing complex underlying behavioral phenomena. Agent-based models (ABMs) have powerful advantages for causal modeling: unlike traditional causal estimation approaches which often result in “one best” causal structure that is learned, two properties of ABMs – equifinality (the ability of different sets of conditions or model representations to yield the same outcome) and mutlifinality (the same ABM might yield different outcomes) – can be exploited to learn multiple diverse “plausible causal models” from data. Causal ABMs leverage the power of machine learning to learn non-linear and sometimes non-closed form relationships between variables. In this tutorial, we will show an illustrative example of how to build a Causal ABM and how to implement ML methods such as Genetic Algorithms for its estimation.
Prerequisites: Python, basic knowledge of causal inference, basic understanding of evolutionary algorithms
Tutorial 5A - "Anomaly Detection"
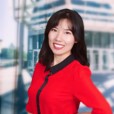
July 11, 2024 | 8:30 AM – 10:00 AM (part 1) & 10:30 AM – 12:00 AM (part 2)
Abstract: In today’s complex business landscape, organizations and marketplaces face numerous challenges in maintaining trust and mitigating risks. Anomaly detection has emerged as a powerful tool to address these concerns effectively. This tutorial aims to provide participants with a comprehensive understanding of the key concepts and techniques involved in anomaly detection and their practical applications in high-stakes domains such as communication surveillance, transaction monitoring, anti-money laundering, and insider trading detection.
Tutorial 6A - "Scoring Strategically: Application to Finance"
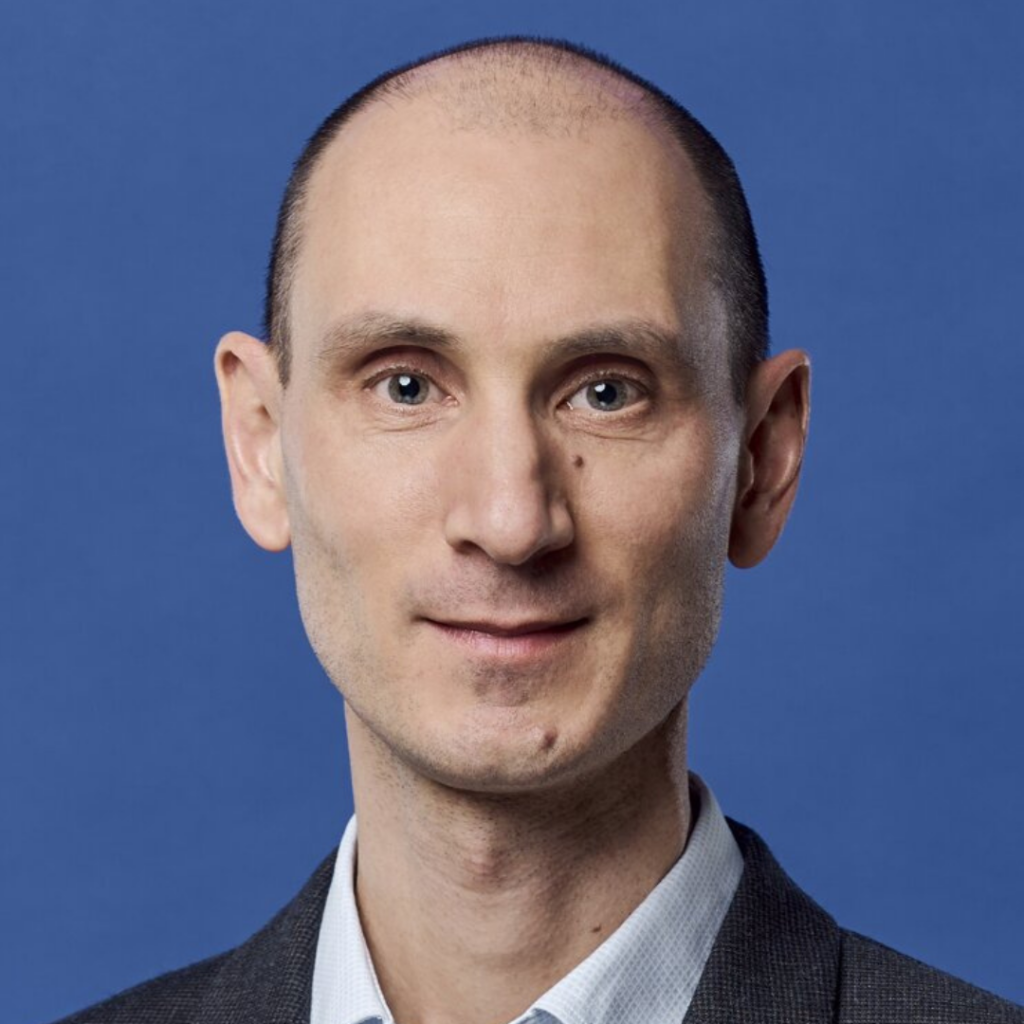
July 11, 2024 | 2:00 PM – 3:30 PM (part 1) & 4:00 PM – 5:30 PM (part 2)
Abstract: This tutorial starts with a brief introduction of fintech lending and the use of credit scoring in credit markets. The main part of the tutorial is an interactive game in which participants play the role of a fintech lender. Context: Banks increasingly use alternative data and machine learning to screen customers and set interest rates. For example, a lender using digital footprints to predict loan default will have a competitive edge over traditional lenders. However, there are important pitfalls to avoid when using alternative data and machine learning to score consumers, such as the winner’s curse and discrimination. This tutorial and its interactive game provide an introduction to these issues.
Track B – Theory and Methods of AI
Tutorial 1B - "Learning on Messy, Tabular Data"
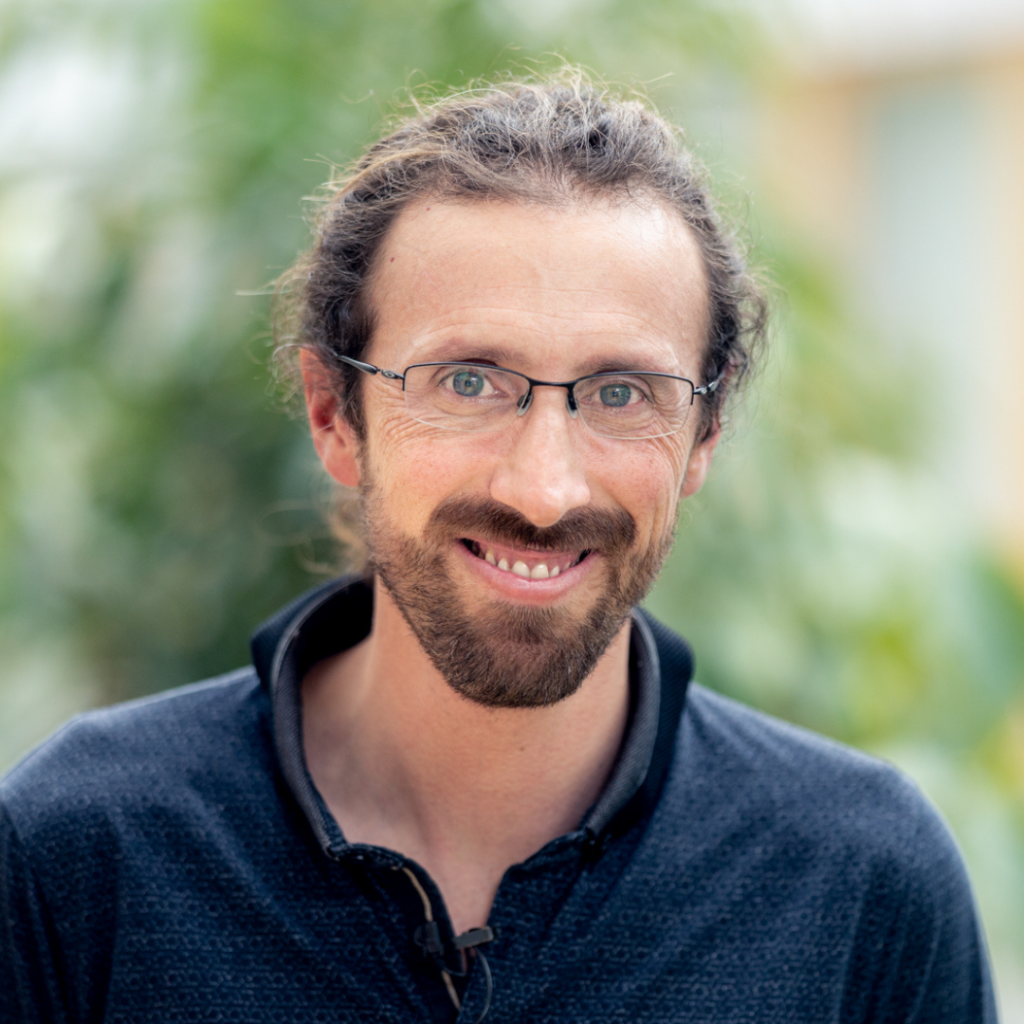
July 8, 2024 | 11:00 AM – 12:30 AM (part 1) & 1:30 PM – 3:00 PM (part 2)
Abstract: Many if not most data science projects are run on tabular data: data from one or multiple tables with columns of diverse nature. Tabular data comes with its own challenges: many entries are of discrete nature (categories or entities), entries may be missing, the data may need to be enriched by joining multiple tables. Additional data-integration challenges arise when the tables are assembled across different sources and come with different conventions. In this lecture I will present various machine-learning methods dedicated to such data. I will illustrate these methods with example using the skrub and scikit-learn Python packages.
Tutorial 2B - "Self-supervised learning in computer vision and medical imaging"
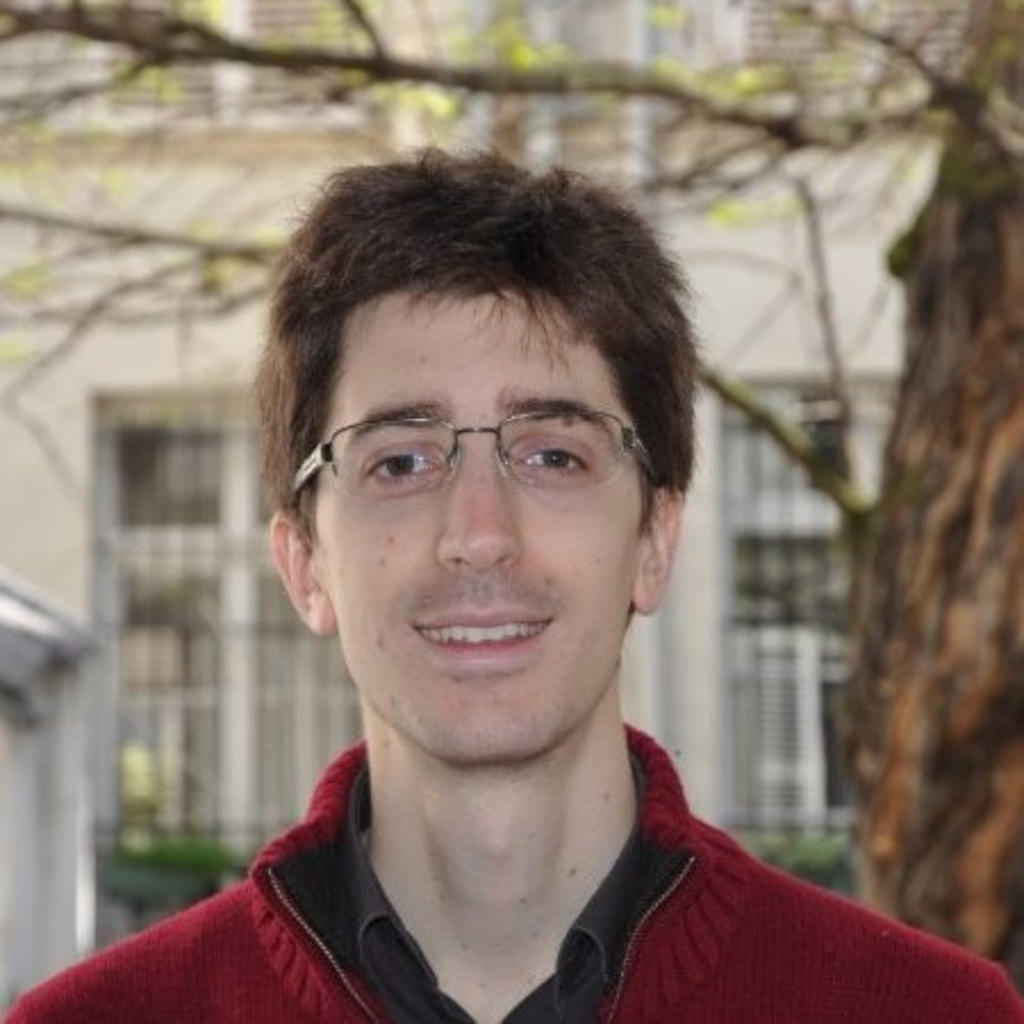
July 9, 2024 | 8:30 AM – 10:00 AM (part 1) & 10:30 AM – 12:00 AM (part 2)
Abstract: Many tasks in Computer Vision and Medical Imaging, such as object detection, image classification, or semantic segmentation, have reached astonishing results in the last years. This has been possible mainly because large (N > 10^6) and labeled data-sets were available. However, in many applications there is a lack of large datasets and/or annotations can be costly, time-consuming and difficult to obtain. Furthermore, it has been recently shown that transferring representations learnt on large datasets, such as ImageNet, is useful only when there is a high visual similarity between pre-training and target domains, namely a small domain gap. To this end, several self-supervised pre-training strategies have recently emerged. They leverage annotation-free pretext tasks to provide surrogate supervision signals for feature learning. These methods can be trained on large, unannotated datasets and then transferred to small, labeled datasets. In the first part of this tutorial, you will learn the most important and used self-supervised strategies for computer vision and medical imaging. In particular, we will study thoroughly contrastive learning using a geometric approach. In the second part, you will test the described methods on both toy exemples and real data using Pytorch.
Tutorial 3B - "Differential Privacy and its trade-off with Fairness and Causality Discovery"
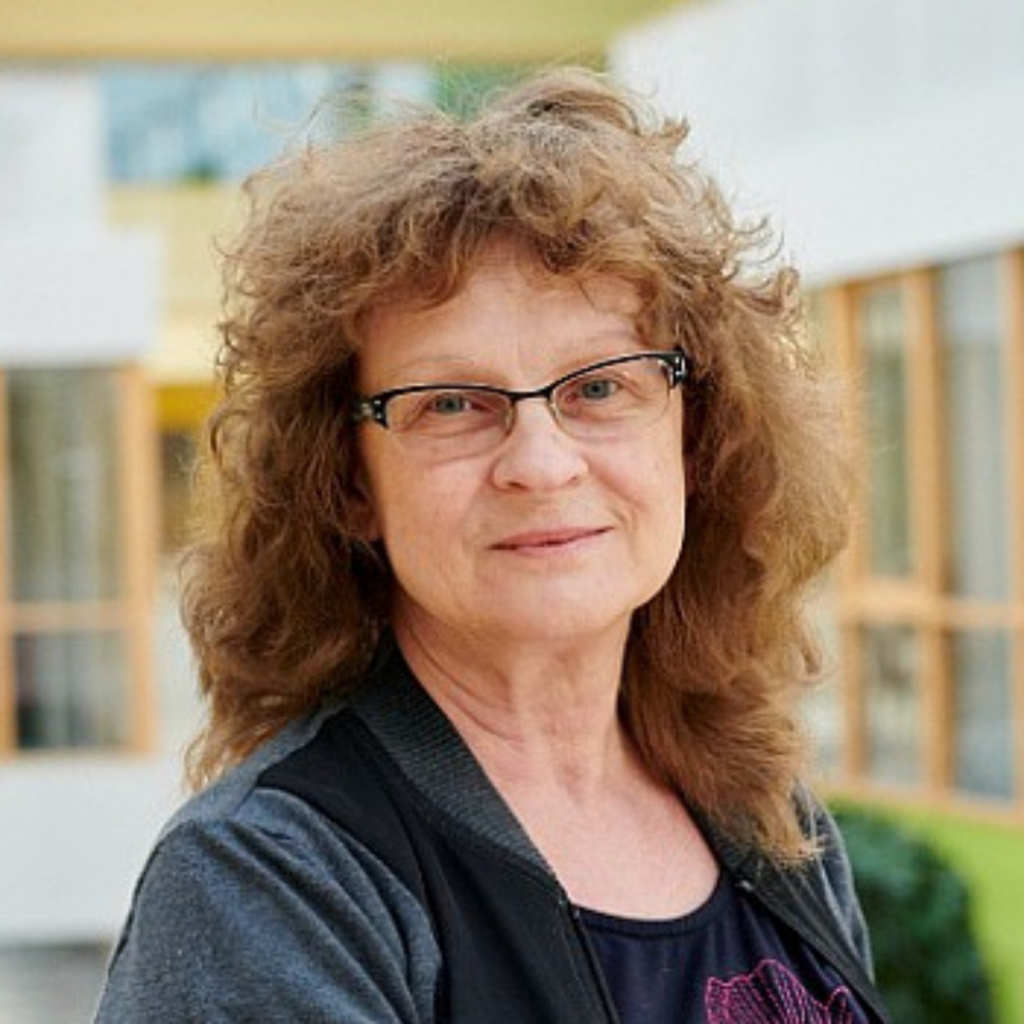
July 9, 2024 | 2:00 PM – 3:30 PM (part 1) & 4:00 PM – 5:30 PM (part 2)
Bio: Catuscia Palamidessi is Director of Research at INRIA Saclay (since 2002), where she leads the team COMETE. She has been Full Professor at the University of Genova, Italy (1994-1997) and Penn State University, USA (1998-2002). Palamidessi’s research interests include Privacy, Machine Learning, Fairness, Secure Information Flow, Formal Methods, and Concurrency. In 2019 she has obtained an ERC advanced grant to conduct research on Privacy and Machine Learning. She has been PC chair of various conferences including LICS and ICALP, and PC member of more than 120 international conferences. She is in the Editorial board of several journals, including the IEEE Transactions in Dependable and Secure Computing, Mathematical Structures in Computer Science, Theoretics, the Journal of Logical and Algebraic Methods in Programming and Acta Informatica. She is serving in the Executive Committee of ACM SIGLOG, CONCUR, and CSL.
Tutorial 4B - "An Introduction to Diffusion Models"
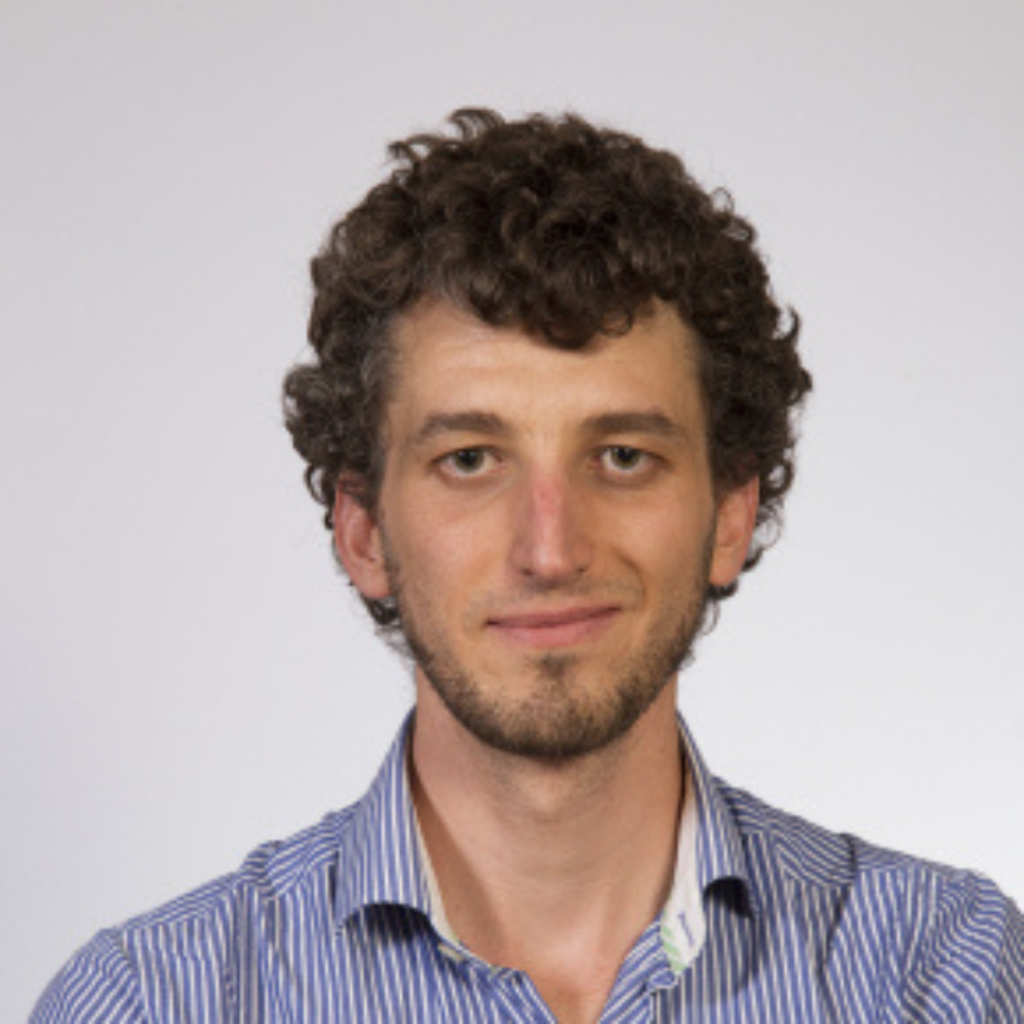
July 10, 2024 | 8:30 AM – 10:00 AM (part 1) & 10:30 AM – 12:00 AM (part 2)
Abstract: In this tutorial, we will present the recent state-of-the-art method for generative modeling: diffusions models. We will study their theoretical aspects (Time reversal of stochastic processes, …) and practical implementation. The goal of the tutorial is to give every attendant the necessary tools to experiment with simple diffusion models both mathematically and practically. If time permits, we will also cover some extensions of diffusion models and discuss their links with other areas of mathematics such as Optimal Transport.
Tutorial 5B - "Empowering Distributed AI: A Deep Dive into Federated Learning"
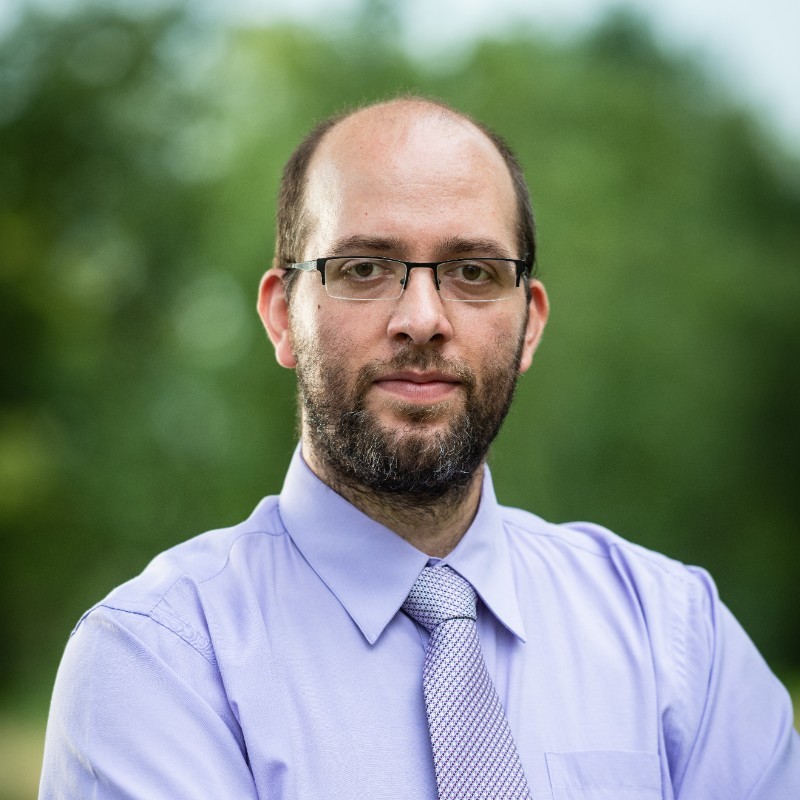
July 11, 2024 | 8:30 AM – 10:00 AM (part 1) & 10:30 AM – 12:00 AM (part 2)
Bio: Federated Learning (FL) represents a paradigm shift in the realm of machine learning, enabling the development of robust AI models without centralizing data. This three-hour tutorial provides an in-depth exploration of FL. We begin by introducing the fundamental concepts of Federated Learning, followed by a detailed explanation of the foundational FedAvg algorithm, which aggregates locally trained models to create a global model.
Next, we delve into the economic perspectives of FL, highlighting how major industry players can leverage FL for competitive advantage, sometimes even adopting adversarial strategies. We then explore the challenge posed by Byzantine workers—malicious or faulty nodes that disrupt the training process—and examine robust defense mechanisms against such threats.
The tutorial also covers Personalized Federated Learning, discussing methods for identifying and collaborating with agents possessing similar data distributions to enhance model performance. We conclude with a discussion on the bottlenecks in FL, such as communication overhead and privacy concerns, and present cutting-edge strategies to mitigate these issues.
By the end of this tutorial, participants will gain a comprehensive understanding of Federated Learning, its economic implications, and the latest advancements in making FL more secure and efficient, empowering them to apply these concepts to their research and practical applications.
- Prerequisites – linear algebra, probability, python, numpy (PyTorch – optional), background in optimization
- Background documents for FL
Tutorial 6B - "Optimal Transport, Wasserstein distance and variants for Machine Learning"
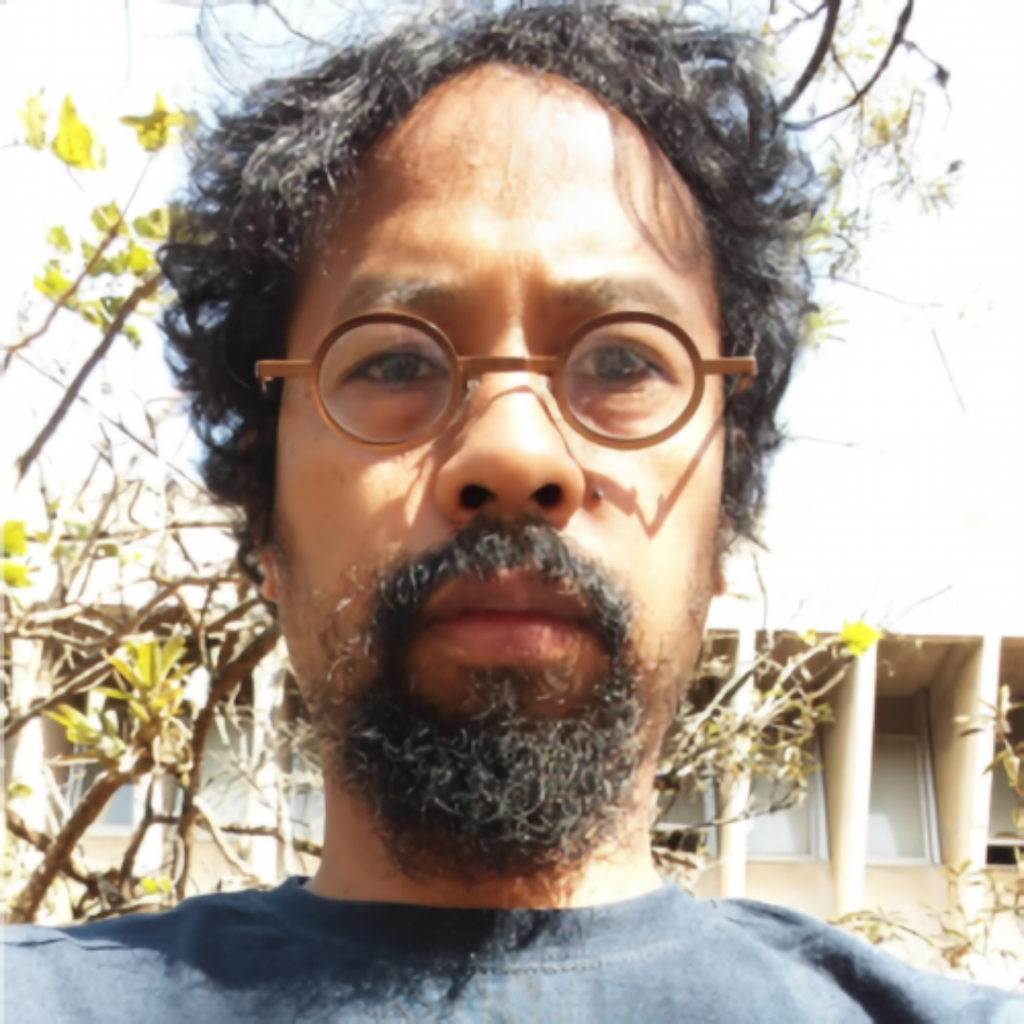
July 11, 2024 | 8:30 AM – 10:00 AM (part 1) & 10:30 AM – 12:00 AM (part 2)
Abstract: This talk provides a introduction to the concepts of Wasserstein distance and Slice Wasserstein distance, along with their applications in the field of machine learning. By exploring the fundamental principles behind these techniques, we will gain a deep understanding of how Wasserstein distance and Sliced Wasserstein can be utilized for tasks such as generative modeling and domain adaptation. Through practical examples and case studies, this tutorial aims to equip researchers and practitioners with the knowledge and tools necessary to leverage the power of these tools in their own machine learning projects.
Keynotes
This year’s Hi! PARIS Summer School will host four Keynote addresses.
More information on each keynote’s topic and speaker can be found below.
Keynote 1 - "The Emerging Science of Benchmarks"
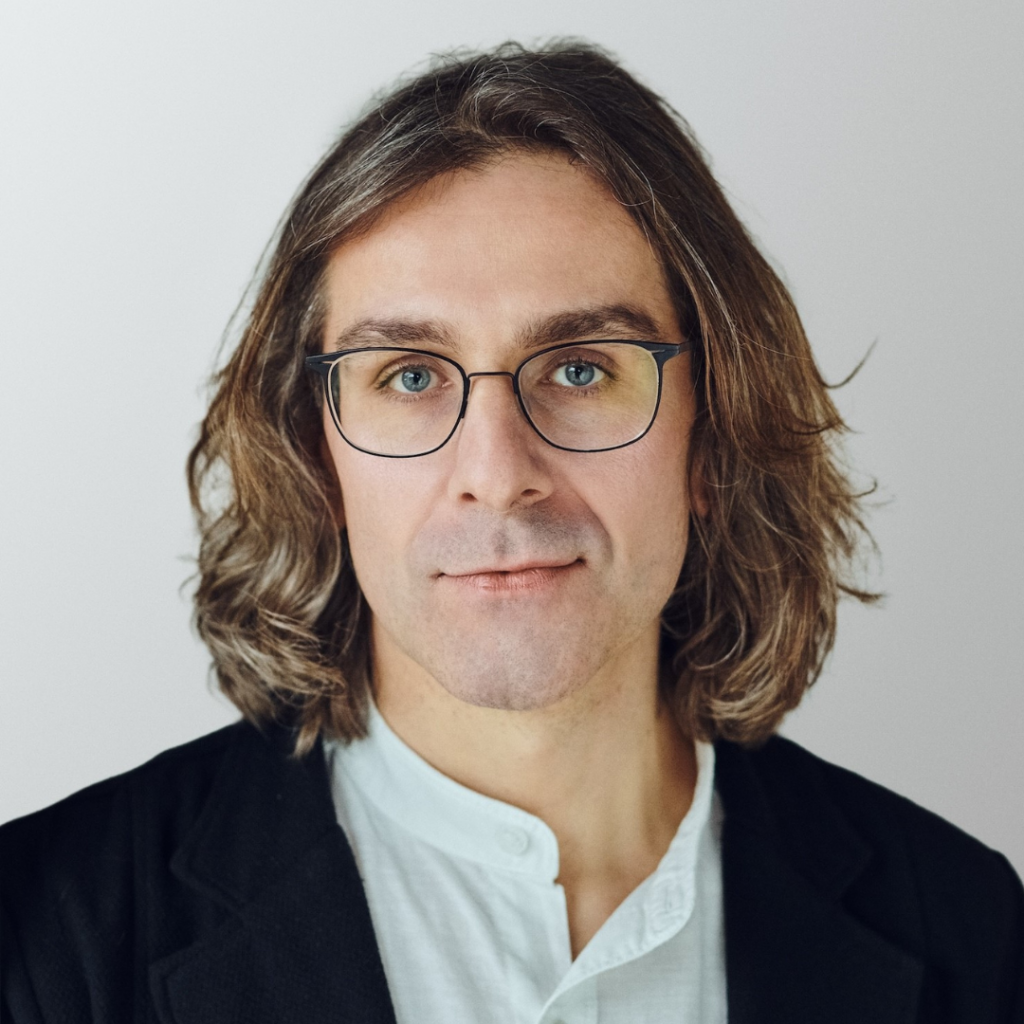
July 8, 2024 | 3:30 PM – 4:30 PM
Abstract: Benchmarks are the keystone that hold the machine learning community together. Growing as a research paradigm since the 1980s, there’s much we’ve done with them, but little we know about them. In this talk, I will trace the rudiments of an emerging science of benchmarks through selected empirical and theoretical observations. Specifically, we’ll discuss the role of annotator errors, external validity of model rankings, and the promise of multi-task benchmarks. The results in each case challenge conventional wisdom and underscore the benefits of developing a science of benchmarks.
Bio: Hardt is a director at the Max Planck Institute for Intelligent Systems, Tübingen. Previously, he was Associate Professor for Electrical Engineering and Computer Sciences at the University of California, Berkeley. His research contributes to the scientific foundations of machine learning and algorithmic decision making with a focus on social questions. He co-authored Fairness and Machine Learning: Limitations and Opportunities (MIT Press) and Patterns, Predictions, and Actions: Foundations of Machine Learning (Princeton University Press).
Keynote 2 - "Artificial Intelligence and the Public Good Most"
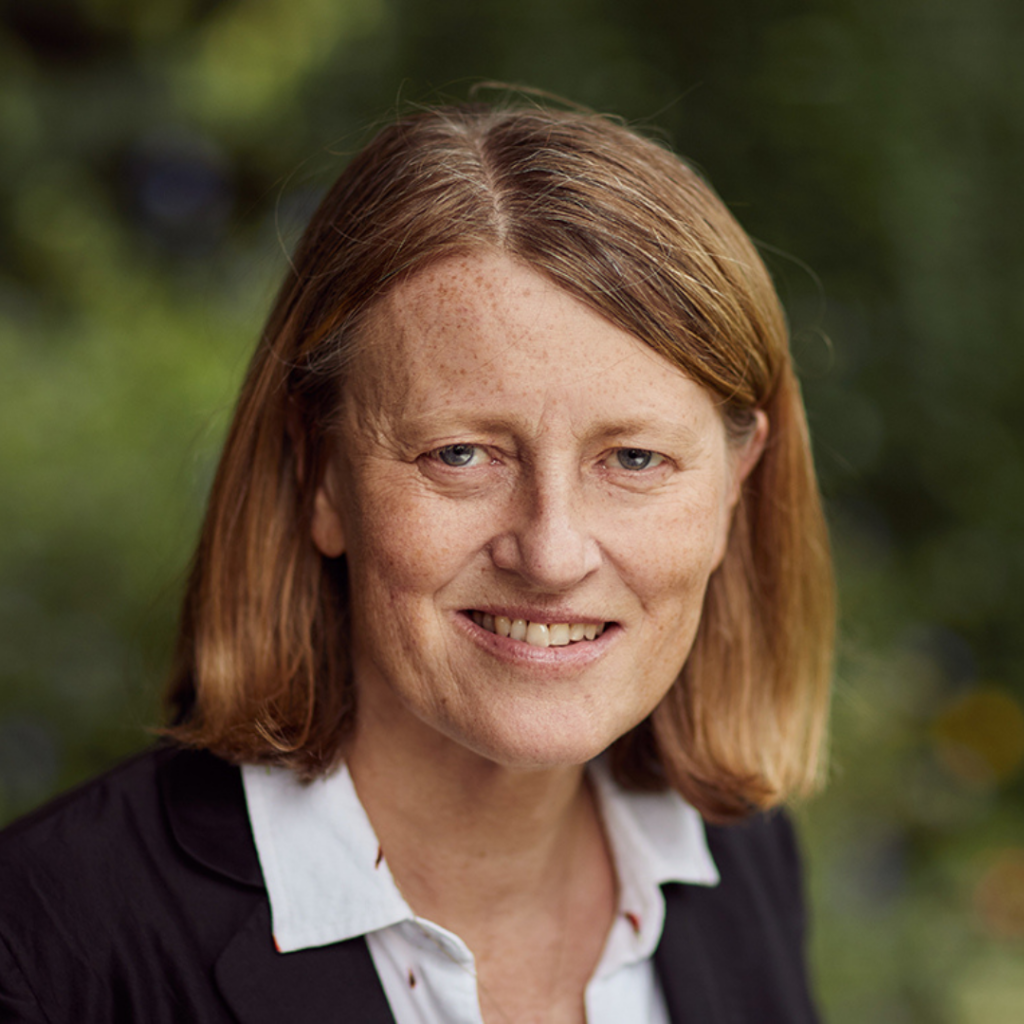
July 9, 2024 | 1:00 PM – 2:00 PM
Abstract: Artificial Intelligence is developed by and for the private sector. This talk will focus on what can happen when we think about AI from a public sector perspective. How can AI be used to improve policymaking, public services and governance? What are the ‘wicked’ public policy problems that AI might help to solve? Drawing on research underway at the Alan Turing Institute for Data Science and AI in the UK, the talk will explain the tasks for which data science and AI are particularly suited. These technologies can bring transformative capabilities to government’s information regime, automate tranches of activity and change administrative logics both vertically and horizontally. Government could be more effective, prescient, productive and citizen-centred than ever before. However, as these technologies become embedded across every market and area of society, they also pose new challenges to governance, public safety and democracy itself, particularly in relation to the power of Silicon Valley firms to shape the information landscape, and these challenges will also be discussed.
Bio: Helen Margetts is Professor of Society and the Internet and Professorial Fellow at Mansfield College. She is a political scientist specialising in the relationship between digital technology and government, politics and public policy.
Since 2018, Helen has been Director of the Public Policy Programme at The Alan Turing Insitute, the UK’s national institute for data science and artificial intelligence. The programme works with policy-makers to research and develop ways of using data science and AI to improve policy-making and service provision, foster government innovation and establish an ethical framework for the use of data science in government. The programme comprises over 90 research projects involving 55 researchers across 20 universities, working with over 100 public sector agencies at national, regional, local and international levels of governance.
Keynote 3 - "AI for the real world - Some challenges"
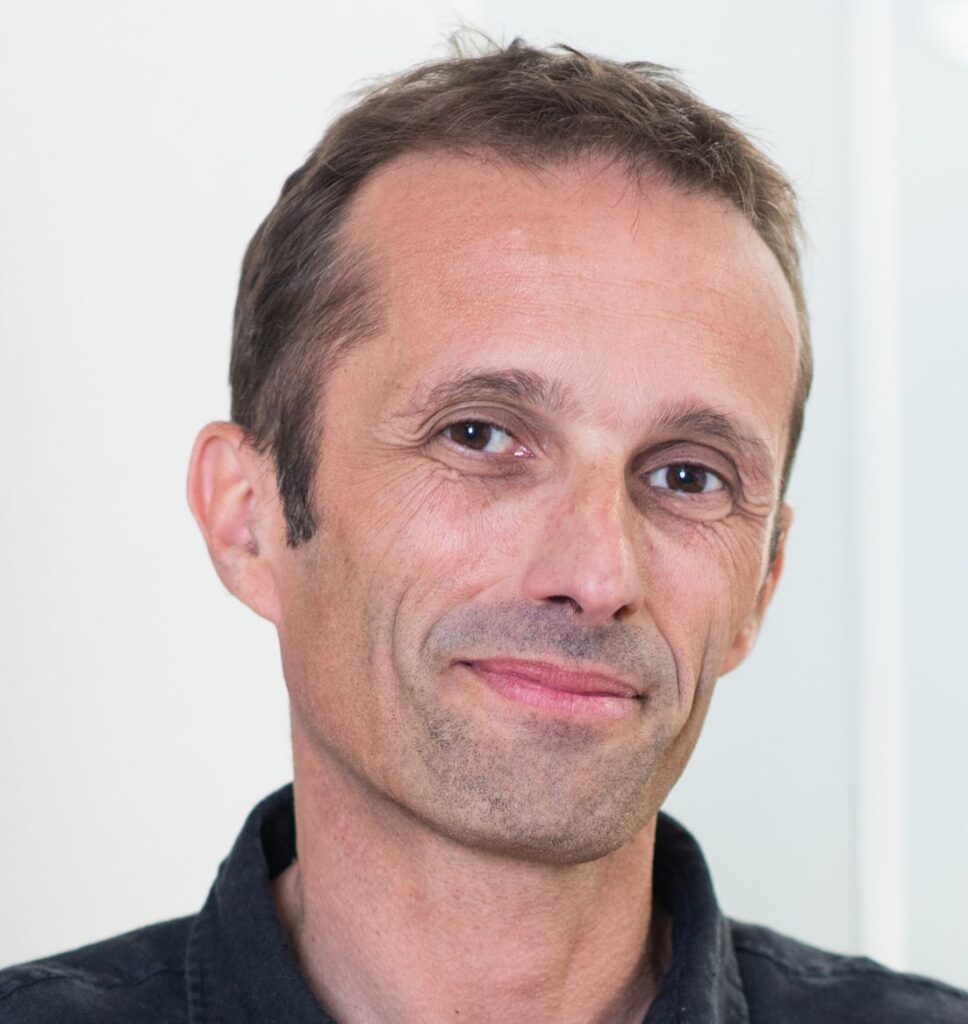
July 10, 2024 | 1:00 PM – 2:00 PM
Abstract: Modern AI is reshaping our digital lives and many industries (including century-old ones). This comes with a variety of key challenges such as scalability, efficiency, reliability, safety and interpretability. I will touch upon some of these challenges, based on research projects across different fields of application, from content creation to autonomous driving. How to tap into raw unannotated data, how to leverage foundation models or world knowledge, how to predict and improve robustness of models for the open world are some of the questions that will be discussed, in the context of visual models. I will conclude with some research directions toward more capable and practical foundation models.
Bio: Patrick Pérez is CEO at Kyutai, a non-profit open-science AI lab, based in Paris. Prior to this, Patrick was at Valeo as VP of AI and Scientific Director of valeo.ai (2018-2023), and with Technicolor (2009-2018), Inria (1993-2000, 2004-2009) and Microsoft Research Cambridge (2000-2004) as research scientist. His research interests lie in reliable multimodal AI for the benefit of all.
Keynote 4 - "LLMs and the Workplace"
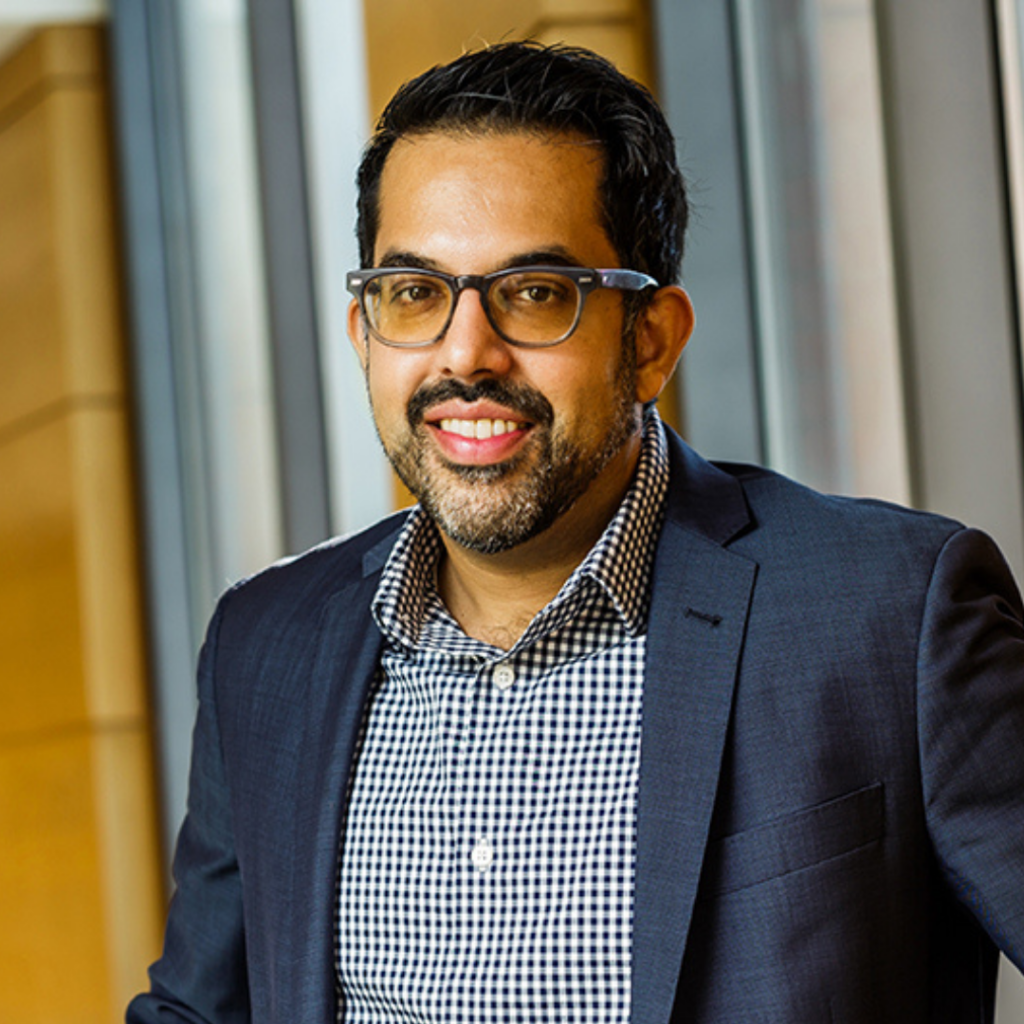
July 11, 2024 | 1:00 PM – 2:00 PM
Abstract: This talk will discuss the opportunities and challenges that arise as Large Language Models (LLMs) and other forms of Generative AI enter the workplace. It will discuss which issues with LLMs are likely to resolve in the short term, as well as which are likely to persist. We will also discuss how jobs might be transformed around these technologies, with an eye towards some future research directions.
Bio: Prasanna (Sonny) Tambe is an Associate Professor of Operations, Information and Decisions at the Wharton School at the University of Pennsylvania. His research focuses on the economics of technology and labor. Recent research projects focus on 1) understanding how firms compete for software developers, 2) how software engineers choose technologies in which to specialize, and 3) how AI is transforming HR management.
Much of this research has uses Internet-scale data sources to measure labor market activity at novel levels of granularity. His published papers have analyzed data from online job sites and other labor market intermediaries that generate databases of fine-grained information on workers’ skills and career paths or on employers’ job requirements. He is a co-author of “The Talent Equation: Big Data Lessons for Navigating the Skills Gap and Building a Competitive Workforce,” published by McGraw Hill in 2013.
Academic Round Table
July 10, 2024 | 2:00 PM – 3:30 PM
The Academic round table will be an opportunity for the audience to learn about the views and research of four leading academic scholars from around the globe, on the opportunities and challenges with Generative AI.
The panel will be moderated by Hi! PARIS scientific co-director and Prof. Gael Richard from Télécom Paris, France. After an opening introduction by the moderator, each of the panel members will be invited to provide a 5-minute presentation of their research in relation to the evolving landscape of Generative AI. This will be followed by a moderated and interactive discussion on the topic to identify future areas of interest for researchers and practitioners. The audience will have the opportunity to ask their questions to the panelists, which are expected to stimulate an discussion on the subject.
Academic speakers:
- HELEN MARGETTS, University of Oxford
- PATRICK PEREZ, Kyutai France
- PRASANNA (SONNY) TAMBE, Wharton, University of Pennsylvania
- MARIE-PAULE CANI, Ecole polytechnique (Hi! PARIS Fellow)
Industry Round Table
July 8, 2024 | 8:45 AM – 10:30 AM
“Opportunities and Challenges with Generative AI”
The Industry round table is composed of representatives from Hi! PARIS Corporate Donors. This event is an opportunity for the audience to learn about Generative AI initiatives being taken by each of the participating companies.
After an opening introduction by the panel moderators, each of the industry panel members will be invited to provide a 5-minute presentation. This will be followed by a jointly moderated session, led by Prof. Aluna Wang and Prof. Matthew Yeaton from HEC Paris, to identify areas of practical interest that can spawn impactful research. The audience will have the opportunity to ask their questions to the panelists. The industry panel will be an interactive event with an opportunity to open communication channels for further research opportunities between the industry and academia.
Practical Research Tips Sessions by the Hi! PARIS Engineering Team
Awais SANI
Senior Machine Learning Engineer1- Learn how to develop your own Python package!
2- Exploring Machine Learning Deployment from beginners to advance level!
Poster Session & Poster Award
Posters will be displayed in HEC Paris Campus from Poster session on Day 2 (Tuesday 9 July, 5:30-6:30pm) for presentation to Poster award on Day 4 (Thursday 11 July, 5:30-6:30pm).
Please note. Posters must be printed by your own means. There will be no printing on site.
Format. The preferred format of the poster is A0 paper, portrait mode (height : 119 cm, width : 84 cm). We will provide you with pins or with tape to hang your poster on the wall.
Guidelines. For Your Convenience, see above some guidelines for poster presentation borrowed from the ICML Conference.
There are many great guides to making accessible and inclusive talks and posters; we advise everyone to consider all the points made in the RECSYS guidelines, the ACM guide, and the W3C guide.
We would like to highlight the following items:
- Keep your posters clear, simple, and uncrowded. Use large, sans-serif fonts, with ample white space between sentences and paragraphs. Use bold for emphasis (instead of italics, underline, or capitalization), and avoid special text effects (e.g., shadows).
- Choose high contrast colors; dark text on a cream background works best.
- Avoid flashing text or graphics. For any graphics, add a brief text description of the graphic right next to it.
- Choose color schemes that can be easily identified by people with all types of color vision and do not rely on color to convey a message (see How to Design for Color Blindness and Color Universal Design for further details).
- Use examples that are understandable and respectful to a diverse, multicultural audience.
You can find an example of good poster and another example of a poor poster here: https://guides.nyu.edu/posters
Social Events
Two social events are schedules as part of the Hi! PARIS Summer school 2024:
- Day 1 (Monday 8 July, 6:00-7:00pm) – Opening welcome cocktail.
- Day 3 (Wednesday 10 July, 6:00-9:00pm) – Cocktail at HEC Paris Le Château
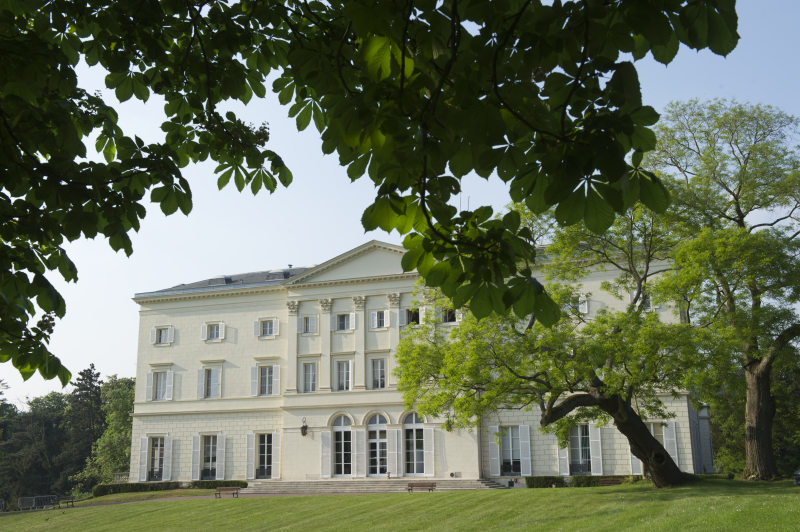