Hi! PARIS Reading groups “Diffusion models for image generation”
The Hi! PARIS reading groups propose to study a topic using scientific articles on a theoretical and a practical point of view. The reading groups are opportunities of interaction between our corporate donors and our affiliates academic teams around selected topics of interest.
Each Edition is planned for 2-4 sessions presenting one topic by the mean of 3-4 research papers. For each session: presentation of mathematical models and theoretical advances by a researcher + simulations with a Python notebook by an engineer.
Registration
Please register to the event using your professional email address to get your personal conference link. Please do not share your personalised link with others, it is unique to you. You will receive an email regarding your registration status.
Diffusion models for image generation
This reading group is devoted to recent diffusion models for image generation. These generative models are based on latent random variables and aim at sampling from highly complex probabilities distributions via a sequence of distributions which transform noise into the distribution of the data. They have many applications in image processing such as image denoising, inpainting, super resolution or image generation. Through three recent papers, we will review the principle of these generative models, how to train them and some challenges related to particular applications.
Session 1/4
Tuesday 27 February, 2024 – 2.00-3.30pm (Online) ** NEW DATE **
- Speaker: Gabriel Cardoso, École polytechnique – IP Paris
- Program: Generative modeling by estimating gradients of the data distribution – Denoising Diffusion Implicit Models.
In this talk we will consider two of the first papers of what is now called Score based generative model. We will begin by “Generative modeling by estimating gradients of the data distribution.”, where for the first time the idea of sampling backwards from a sequence of diffused versions of the data is explored and that for the first time beat GAN’s in image generation tasks. We then will look at DDIM (Denoising Diffusion Implicit Models), which is a much more efficient sampler to sample backward from the same sequence of diffused distributions. We will compare the tradeoff obtained by sampling from the two algorithms.
Paper:
- NCSN: Song, Y., & Ermon, S. (2019). Generative modeling by estimating gradients of the data distribution. Advances in neural information processing systems, 32.
- DDIM: Song, J., Meng, C., & Ermon, S. (2020, October). Denoising Diffusion Implicit Models. In International Conference on Learning Representations.
Session 2/4
Tuesday 12 March, 2024 – 2.00-3.30pm (Online)
- Speaker: Stéphane Latuilière – Marlène Careil, Télécom Paris – IP Paris.
- Program: Score-Based Generative Modeling through Stochastic Differential Equations Zero-shot spatial layout conditioning for text-to-image diffusion models.
- Paper: https://arxiv.org/abs/2306.1375
Session 3/4
Tuesday 9 April, 2024 – 2.00-3.30pm (Online)
- Speaker: Vicky Kalogeiton – Xi Wang, Ecole polytechnique – IP Paris.
- Program: Adding Conditional Control to Text-to-Image Diffusion Models: ControlNet is a NN structure to control diffusion models (including Stable Diffusion) by adding extra conditions, a game changer for AI Image generation.
Unlike prior work that could not efficiently or simply instruct an AI model which parts of an input image to keep, ControlNet addresses this by introducing a method to enable Stable Diffusion models to use additional input conditions that tell the model exactly what to do.
- Paper: https://arxiv.org/abs/2302.05543
Session 4/4
Tuesday 14 May, 2024 – 2.00-3.30pm (Online)
- Speaker: Yazid Janati, École polytechnique – IP Paris.
- Program: Score-Based Generative Modeling through Stochastic Differential Equations.
We will present the basics of denoising diffusion probabilistic models which are the heart of generative AI revolution. We will detail the model then discuss theoretical and practical aspects.
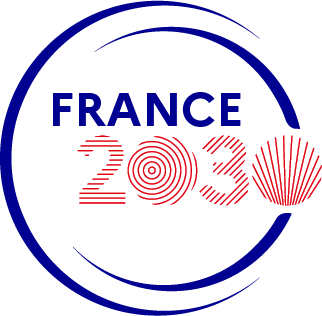
This work has benefited from a government grant managed by the ANR under France 2030 with the reference “ANR-22-CMAS-0002”.